All published articles of this journal are available on ScienceDirect.
Single-cell RNA Sequencing: Current Progresses and Future Perspectives
Abstract
During the last few years, advancements in the areas of biochemistry, the science of the material world, engineering, and computer-aided testing have been directed toward the development of high-throughput tools for profiling information encoded in genes. Single-cell RNA sequencing (scRNA-seq) tools are capable of examining sequence data from individual cells, revealing population variety and allowing exploration of cell conditions and transformations with extreme resolution. These tools can potentially identify cell subtypes or gene expression fluctuations that are obscured in mass sequencing processes, which provide population-averaged evaluations. However, a major disadvantage of this tool is the inability to pinpoint location-related details of the RNA transcriptome, as this requires tissue detachment and cell isolation. Location-based transcript determination represents an advancement in medical biotechnology, as it can identify molecules, such as RNA datasets, in their intact physical placement within tissue segments with spatial context at the single-cell scale. This capability is highly advantageous compared to traditional single-cell sequencing techniques. These approaches offer valuable insights into various sub-disciplines of the biomedical field, including neurology, embryology, carcinoma studies, immune cell investigation, and histological activities. This review primarily focuses on single-cell sequencing methods, technology development, observed challenges, different expression data analysis mechanisms, and their applications in various areas, such as cancer research, microbes, the central nervous system, reproductive organs, and immunobiology. It underscores the importance of sequencing tools at the single-cell level for characterizing highly dynamic individual cells.
1. INTRODUCTION
During the last few years, advancements in the areas of biochemistry, the science of the material world, engineering, and computer-aided testing have been directed towards the advancement of high-throughput tools for the purpose of profiling information encoded in genes and sequencing figures from different biological specimens. Technologies are now capable of sequencing many fragments of DNA in parallel, such as RNA sequencing, enabling an intense and full understanding of complex events involved in an organism, including the whole organism development process, the re-establishment of damaged tissue into normal structure and function, and carcinogenesis [1]. The recent advancements in genetic testing and sequencing technology have encouraged the progression of applying sequencing-supported tools for exploring genomic heterogeneity and fluctuations within a body system [2]. Currently, there are numerous techniques used for gene expression profiling. Among these techniques, the RNA-sequencing mechanism (RNA-seq) permits transcript investigation with extraordinary precision and breadth, leading to the discovery of newly identified RNA species and enhancing our understanding of transcriptomic changes [3, 4].
Recently, low-input RNA-sequencing techniques have been developed to be used for studying single-cell characterizations [5]. Single-cell RNA-sequencing (scRNA-seq) tools are capable of examining sequence data from each cell. They reveal the within-population variety and permit the exploration of cell conditions and transformations with extreme resolution. These tools potentially identify cell sub-types or gene expression fluctuations that are obscured in mass sequencing processes, which provide population-averaged evaluations [6, 7].
Investigating every individual cell through global mechanisms has the capability to transform our understanding of full organisms. From that point on, cell ancestry can be traced, and variability within a tissue can be summarized with extraordinary resolution [8]. Exploring cells from tissue at the individual level creates a special scope to assess the interaction between inherent cellular activities and external signals, such as the surrounding circumstances or adjacent cellular interplays, in determining cell destiny. On the other hand, single-cell exploration is highly beneficial in medical centers, helping to investigate how an “outlier cell” may influence the outcome of disease progression [9], medication or anti-microbial resistance [10, 11], and tumor reversion [12]. Moreover, since most cells from life forms are unable to be cultured in external environments (often referred to as “microbial dark matter”), examination at the single-cell level holds the opportunity to discover unrecognized species or governing mechanisms of biotechnological or therapeutic importance [13].
High-efficiency single-cell RNA profiling has enabled extraordinary insight into the cellular heterogeneity of tissues across various life forms. Gene expression analysis using bulk tissue considers and characterizes all the cells in a way that treats identical bodies similarly, thereby ignoring the statistical probability of gene expression [14, 15]. To address issues related to the probabilistic nature of gene expression, single-cell sequencing techniques are highly suitable.
Single-cell sequencing techniques involve the sequencing of the genetic material from each isolated cell to obtain genomic, transcriptomic, or multi-omics data at a single-cell scale, revealing cell population heterogeneity and cellular developmental associations. Ordinary sequencing techniques struggle to dissect the median of numerous cells, cannot explore a small number of cells, and mask important cellular variability information. In contrast to conventional sequencing tools, single-cell sequencing tools are essential for dissecting gene expression variability among individual cells [16]. Differentiating a limited number of cells in terms of quantity and tracing out cell maps are key capabilities of single-cell sequencing techniques. Meanwhile, in 2013, single-cell sequencing techniques were recognized as a significant innovation by being named “Nature Methods” as the yearly innovation [17]. However, previous single-cell sequencing protocols were limited to adoption due to their expensive implementation costs and the considerable effort required to apply the techniques.
The global analysis of single cells has been performed through significant advancements in the sensitivity of scientific instruments and the increasing automation of every step, from specimen preparation to information analysis. This enables the detection of gene expression dynamics at a tiny level within the cell population. High-throughput technologies now allow for the rapid sequencing of the genomes of many single cells in a parallel fashion, generating large amounts of information in short time periods [18]. Alternatively, the proteins expressed can be identified by utilizing techniques, such as fluorescence and mass cytometry [19]. Exploration of messenger RNA in single cells has been initiated through a variety of probe-based techniques, including reporter fusion to fluorescent proteins, fluorescence in situ hybridization (FISH), quantitative real-time PCR (qRT-PCR), and microarrays [20]. Many of these techniques can provide an assessment of the expression variability of multiple genetic materials in a parallel fashion.
Generally, this review primarily focuses on single-cell sequencing methods, technology development, challenges, and their applications in various areas, including cancer research, microbiology, the central nervous system, reproductive organs, and immunobiology. It emphasizes the importance of sequencing tools at the single-cell level for characterizing highly dynamic individual cells. However, single-cell RNA sequencing tools currently face challenges related to skilled workforce and high profiling costs, which limits their usage in transcriptomic studies.
1.1. Single-cell RNA Sequencing
The single-cell RNA sequencing method analyzes the gene expression profiles of individual cells generated from homogenous and heterogeneous population sources. This method isolates individual cells, typically by techniques of encapsulation or flow cytometry, and then amplifies and sequences the RNA transcripts from each cell separately. This high-resolution mechanism allows researchers to characterize cell types, states, and subpopulations. Single-cell RNA sequencing techniques can also reveal cellular heterogeneity and rare cell populations that might be masked in bulk RNA-seq data methods. The single-cell RNA sequencing method applies to numerous investigations in life science, like comparing gene expression profiles of individual cells. Researchers can investigate and characterize novel or rare cell populations, as well as further refine known cell types and study cellular differentiation, lineage tracing, and developmental trajectories in different organisms, providing important information on the regulatory pathways controlling cellular fate decisions [21].
2. FROM BULK TO SINGLE-CELL TRANSCRIPTOMIC DISSECTION
Transcriptomic examination at the individual cell resolution level was initiated around 20 years ago by Norman Iscove, who utilized exponential amplification techniques of single-cell cDNAs via polymerase chain reaction. Additionally, James Eberwine pioneered the straightforward amplification of cDNAs through T7 RNA polymerase-based transcription outside the living body. The mechanisms mentioned above have accelerated the exploration of deeper molecular systems involved in the developmental process and the functioning mechanisms of the vertebrate nervous system, particularly because these cells are among the most varied class of cells. In such cases, transcriptomes at the single-cell or lower-level determination in a prolonged axon can provide descriptive insights [22, 23]. Subsequently, the implementation of mass-produced, accessible, high-density DNA microarray chips accelerated the advancement in individual cell microarrays [24].
The latest findings have demonstrated significant cell-to-cell transcriptomic dissimilarities [25]. Even among ancestrally invariant cell clusters, there are significant transcriptomic differences. Consequently, mass assessments can obscure fundamental cellular variability [26] and generate averaging biases, thereby obscuring the reality of gene expression dynamics among cell populations in scientific investigations.
So far, almost all transcriptomic analyses are conducted at a “population level,” consistently resulting in mean transcriptomes of numerous cells. However, under certain conditions, such as with stem cells, malignant tumor cells, and other scarce populations, there is difficulty in obtaining sufficient material to perform determinations at such a degree. Furthermore, mass techniques are unable to investigate the ultra-fine but biologically significant variability between apparently similar cells. Despite each vertebrate animal cell being estimated to accommodate approximately 105-106 expressed mRNA genes [27], the relative percentage of various transcriptomic groups in a population varies significantly [28]. Moreover, a quantifiable characterization in yeast [29] has proven that most messenger RNAs are available in a small number of less than 5 transcriptome duplicates within each cell, and the majority long non-coding RNAs are present surprisingly in less than 0.5 duplicates per cell. Moreover, in certain bacteria, the mean copy number of messenger-RNA in Escherichia coli is 0.4 per cell [30]. Fig. (1) presents the workflow for mass and single-cell RNA sequencing process in relation to data generated between them.
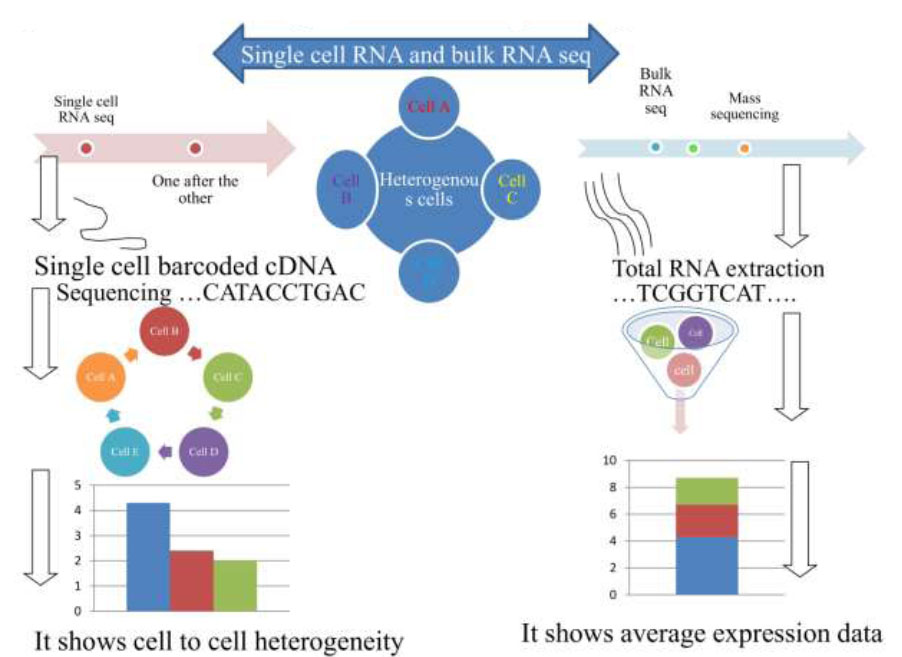
Mass and single-cell RNA sequencing technique procedures.
The single-cell RNA sequencing method has been proven effective in characterizing multiplex, varied cell populations, allowing for a comprehensive understanding of population composition and the discovery of new subtypes and rare cell types [31, 32]. In situations involving dynamic processes, the reorganization of cell paths from single-cell profiling data has facilitated the investigation of short-term, intermediate cell states and has helped to identify key regulatory genes [33].
Additionally, the single-cell RNA sequencing method also holds great promise for understanding probabilistic transcriptional bursting and deciphering gene regulatory systems. However, deriving system hypotheses from single-cell RNA sequencing data is computationally challenging and difficult to confirm. Inferred system models should, therefore, be rigorously characterized and practically validated where possible. Moreover, a particular mRNA may be expressed at various scales within a cell population either due to deterministic reasons as it is part of a regulated cellular process or due to random fluctuations in expression levels among cells, a phenomenon also known as transcriptional noise, which should not be overlooked as insignificant because it has significant implications in cell fate determination [34].
RNA-seq of single cells enables researchers to uncover the unique characteristics of biological cells at individual levels within complex tissues and organ systems. It also aids in understanding the response of cellular subpopulations to environmental conditions. RNA-seq has facilitated the initial determination of the extent of transcriptomic variability, encompassing both coding and non-coding RNAs, across a genome-wide scale. The techniques of single-cell RNA sequencing will similarly assist in deciphering time-related transcriptional networks during transitional mechanisms [7] or when cells are exposed to the external environment [34-37], each of which may be obscured at a population scale (Table 1).
3. PROGRESS IN SINGLE-CELL RNA PROFILING TECHNIQUES AND INNOVATION
Progress in the field of life science investigation continues to deepen, with researchers working with single-cell RNA sequencing tools making advancements in minimizing dissecting costs. This progress enables researchers to delve deeper into the molecular workings of individual cells, advancing our understanding at the cellular level. As proposed by other findings [35], a single-cell integrative label sequencing tool (SCI-seq) can simultaneously construct multiple single-cell information libraries and analyze heterogeneity in body cell copy numbers. This method maximizes the number of tissue cells characterized while minimizing the cost of library preparation, providing significant advantages for the analysis of body cell heterogeneity.
No. | Bulk RNA Seq | Single-cell RNA Seq |
---|---|---|
1. | Measures the average gene expression across heterogeneous cells | Analysis of gene expression profiles of individual cells |
2. | RNA from many different cell types is extracted | RNA from individual cells extracted |
3. | All RNA transcripts pooled together | Each RNA transcript is investigated separately |
4. | All sequenced together | Sequenced individually |
5. | Low-resolution approach | High-resolution approach |
6. | Mask the important information available at a scale cellular level | Can show cellular heterogeneity and rear cell populations |
7. | Gene expression profiling | Study cellular heterogeneity |
8. | Transcriptome annotation | Cell type identification and characterization |
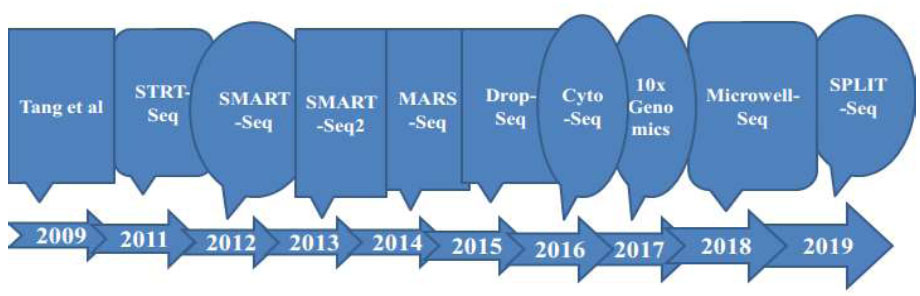
Timeline of improvements in single-cell sequencing methods as a base for technology progressions to the next level.
According to an investigation [36], a new single-cell whole-genome amplification technique has been innovated that enables the examination of copy number variations (CNV) at the kilobase level and further allows for the efficient characterization of mutations in various diseases. Another tool developed [37] is mainly based on sequencing single cells by a parallel method known as scCOOL-seq. This technique enables the concurrent detection of individual cell genomic conditions or nuclear micro-environment organization, copy number heterogeneity, repetition of chromosome sets, and analysis of methylated DNA. It is capable of revealing various activities and forms of genomic conditions and DNA, including heritable epigenetic methylation, where a methyl group attaches to a specific spot on a gene. Other studies [38] designed mechanisms for Topographic Single-Cell RNA Sequencing (TSCS), enabling precise spatial positional data for every individual cell. This method has a high degree of sensitivity to investigate and express the particular nature of each cancer cell's positional information, allowing the study of the occupation and malignant nature of cancer cells. Fig. (2) shows events arranged based on the progression and advancements of single-cell RNA sequencing techniques.
Another mechanism describes a highly efficient and minimally divergent single-cell RNA sequencing technique that utilizes droplet-based microfluidics to isolate, expand, and barcode the genetic material of individual cells. This methodology allows for a broader characterization of genetic material across various cell populations [39]. Additionally, Microwell-seq, developed by another investigator [40], is an advanced and cost-effective single-cell RNA sequencing methodology. It not only enhances the investigation of various single-cell RNA tools but also significantly reduces the cost of examination by employing a series of dimensions analyzed through single-cell RNA sequencing methods encapsulated within oil droplets. The SPLiT-seq tool, developed based on the concept of a cost-effective combined barcode, is capable of reducing the cost of single-cell RNA transcriptomic sequencing to the one-cent level. By further lowering the price threshold for single-cell characterization, this technology becomes more accessible to researchers [41].
Currently, there are no standardized single-cell sequencing techniques, indicating that researchers choose the method they follow based on their study objectives and available options. Based on transcript coverage, single-cell transcriptome sequencing tools currently utilized can be grouped into different types [42]: (i) entire-length transcriptome sequencing mechanism (one example- MATQ-seq [43], SMART-seq2 [44], ICELL8 [45] and SUPeR-seq [46]), (ii) 5′ -end transcriptome sequencing techniques (for instance: STRT-seq ([47]), and (iii) 3′ -end transcriptome sequencing technique (one prototype- Chromium [48] 10X Genomics, Fluidigm C1 [49], Drop-seq [50], in Drop ([51]).
Following an entire-length transcriptomic sequencing method, there is a problem of strength of character, rate, and sequencing price. On the contrary, the main problem of sequencing cDNA is emphasizing either the 5′ or 3-end transcriptome of the DNA, thus lacking the ability to explore allele-related gene expression or alternative splice fashion. Various techniques rely on flow-activated cell sorting (FACS) for arrangement, including MARS-seq, which relies on a large starting volume [52] and becomes impractical when there is minimal starting volume, such as with fine-needle aspiration. Another issue with using the flow-activated cell sorting (FACS) method is the requirement of applying antibody beads that target specific proteins for sorting. This creates challenges when categorizing rare cell subtypes [53]. Thus, each method has its own set of pros and cons that determine the 'depth' of the data collection, which can significantly impact both numerical and biological interpretations [54].
Single-cell RNA sequencing is not a 'one-size-fits-all' method, unlike mass sequencing mechanisms. The depth of analysis can vary depending on the protocols followed, types of cells being characterized, isolation techniques, sequencing methods, and stringency of association during library preparation [55]. The single-cell RNA sequencing technique initially involves the isolation of individual cells from biological samples. However, the capability to capture poses a significant challenge for the single-cell RNA sequencing workflow. Highly sensitive isolation methods and skilled personnel are required to enhance the accuracy of detecting transcriptome expression dynamics. Nowadays, a variety of techniques are available for separating single cells from biological samples, including limiting dilution techniques, flow-activated cell sorting (FACS), micromanipulation, laser capture micro-dissection (LCM), and microfluidics [56-58].
The limiting dilution method employs pipettes to separate targeted cells from heterogeneous populations through the process of dilution. However, a major drawback of using this technique is its limited productivity compared to other methods. Micro-dissection or microinjection is a typical mechanism used to isolate cells from specimens with minimal quantities of cells, such as early-developing embryos or uncultured microorganisms. However, using these methods is time-consuming and has a low throughput. Fluorescence-activated cell sorting (FACS) has been widely utilized to select targeted individual cells but requires large processing volumes (typically >10,000 cells) in suspension solution. However, there are drawbacks associated with FACS, such as slow sample processing speed. Laser capture micro-dissection (LCM) is an advanced technique used to select individual cells, primarily from solid tissue specimens, using a laser mechanism assisted by a computer. The main advantage of LCM is its rapid processing speed of cell samples. However, a significant drawback of this technique is the requirement for optical microscopic investigation to identify single cells within complex tissues, necessitating expertise in cell characterization. Microfluidics is progressively becoming a well-known technique due to its minimal specimen usage, precise fluid control, and low investigation costs. Each of these single-cell isolation procedures has its own importance and demonstrates varying levels of success in terms of isolation efficiency and clarity of the targeted cells [52, 58].
Various scRNA-seq procedures may have their own advantages and disadvantages, and numerous published analyses have compared some of them extensively [59]. A previous analysis illustrated that the Smart-seq2 method can identify a huge quantity of expressed genes than other forms of scRNA-seq tools along with CEL-seq2 [60], MARS-seq [61], Smart-seq [62], and Drop-seq mechanisms [63]. Currently, Sheng et al. demonstrated that an additional full-length transcriptome sequencing method, MATQ-seq, could outperform Smart-seq2 in analyzing genes with minimal occurrence [43]. Compared to 3′-end or 5′-end counting procedures, full-length single-cell RNA sequencing techniques hold extraordinary significance in investigating isoform usage, allelic expression characterization, and RNA editing due to their comprehensive coverage of the transcriptome. Moreover, for studying lowly expressed transcripts that are inevitable, full-length single-cell RNA methods could offer advantages over 3′ sequencing approaches [63].
Particularly, droplet-centered tools (for example, Drop-seq [50], InDrop [51], and Chromium [48]) can predominantly give a huge parallel sequencing of single cells and a minimal sequencing price for each cell in comparison with the entire-transcriptome single-cell RNA sequencing. Thus, droplet-based mechanisms are more reliable for producing numerous quantities of cells to detect the cell sub-populations of sophisticated tissues or cancer specimens. Notably, many single-cell RNA sequencing tools can identify the two polyA+ and polyA− RNAs, like SUPeR-seq [46] and MATQ-seq [43]. These mechanisms are crucial for sequencing long, non-coding RNAs and circular RNAs.
To estimate the technical differences between various types of cells and spike-ins, such as External RNA Control Consortium (ERCC) controls [64], Unique Molecular Identifiers (UMIs) have become widely used in related single-cell RNA sequencing techniques.
RNA spike-ins are RNA transcripts with known sequences and quantities utilized to calibrate the measurements of RNA hybridization assays, such as RNA sequencing. UMIs enable the theoretical enhancement of total molecular counts. Spike-ins are utilized in techniques, such as Smart-seq2 and SUPeR-seq, but are not compatible with droplet-based techniques. However, UMIs are predominantly used in 3′-end sequencing methods (like Drop-seq [50], InDrop [51], and MARS-seq) [61]. As a result, the appropriate single-cell RNA sequencing approach can be selected in experiments based on practical considerations and priorities, including the number of cells to be sequenced and cost considerations.
3.1. Current Progress in Single-cell RNA Sequencing Tools
Cells from organisms were investigated in the 16th century. After numerous developments and the progress of novel techniques, mechanisms emerged in a transition from simple to a more powerful way. While the introductory microscope, designed by Zacharias Janssen and Hans Lippershey in the early 16th century, allowed Robert Hooke and Anton van Leeuwenhoek to observe the initial living cell in the 17th century, the process took nearly two centuries to fully reevaluate cells, regarding them not only as the structural but also the functional unit of life [65]. Preceding this time, a number of investigations and techniques were performed with the desire to achieve a higher-grade sense of perception and to investigate cells within heterogeneous multicellular networks [66].
The initial visionary and practical advancement in single-cell RNA sequencing techniques involved [5] sequencing the transcripts of single blastomeres and oocytes. The idea and tools carried out by this analysis unlocked a novel mechanism for addressing the challenge of scaling up the number of cells and generating agreeably improved RNA sequencing mechanisms feasible for the early period. Above all, prices have been surprisingly minimized while robotization and advancement have been remarkably accelerated. All these procedures have culminated in more developed scRNA-seq mechanisms, yet the fundamental idea of utilizing single-cell RNA sequencing remains unchanged [21].
In the case of humans, single-cell investigation has upgraded detailed awareness of developmental and biological activity in our body systems [67], aging [68], and a number of disease characterizations like tumor development [69]. However, there is an issue in producing universal blueprints for single-cell RNA transcript investigation, given that each method requires the user to make informed judgments to obtain interpretable outcomes. These decisions include selecting specimen types, determining the number of cells and preparation methods, choosing single-cell RNA sequencing techniques and sequencing protocols, and designing computational
Methods | Applications in Single-cell RNA Sequencing |
---|---|
SCI-seq | - Can simultaneously construct multiple single-cell information libraries and analyze heterogeneity in body cell copy number |
scCOOL-seq | - Sequencing single cells by parallel method and analysis of methylated DNA |
TSCS | - Enabling precise spatial positional data for individual cells |
Microwell-seq | - Significantly reduce the cost of examination |
SMART-seq2 | - Enable entire-length transcriptome sequencing mechanisms |
Drop-seq and Chromium | - Can give huge parallel sequencing of single cells |
analysis frameworks to derive insights from single-cell datasets. Essentially, achieving effective single-cell RNA transcriptomic analysis with interpretable data files and relevant scientific results can only be realized through the use of well-defined experimental protocols (Table 2).
3.2. Single-cell Isolation Process and Library Preparation
Variations in RNA transcriptomic and gene expression among individual cells can offer crucial insights into solving problems in tumor biology, neuroscience, stem cell differentiation, immunology, and evolutionary biology. To achieve this, the first step involves isolating individual cells from tissues, which is essential for capturing high-quality single cells from a given body sample. This isolation process ensures the accurate preservation of genomic and biochemical processes occurring within cellular activities, enabling the analysis of specific genomic and molecular operations [70]. Conventional transcriptomic, epigenomic, or proteomic analyses from bulk RNA or DNA specimens can only provide an aggregate of information from tissues or organs, lacking the ability to detect the heterogeneity present among individual cells. To gain a deeper understanding of variations between cells, researchers are turning to the approach of single-cell RNA sequencing analysis, which can provide highly granular datasets essential for precision medicine decision-making. The techniques for isolating single cells and capturing their profiles vary significantly depending on the organisms, tissues, or cell types involved [71].
Before embarking on single-cell investigations, researchers must isolate individual cells from tissue samples. Cell separation can be achieved by selecting entire cells, isolating cell-specific nuclei or organelles, or isolating cells expressing specific marker proteins [72]. The primary purpose of isolating individual cells, particularly through parallel mechanisms, is to ensure that each cell is isolated in a separate reaction mixture. Specifically, all transcriptomes from each individual single cell are uniquely barcoded before being converted into complementary DNAs (cDNA) [21].
Indeed, single-cell RNA sequencing methods have progressively revealed several intrinsic procedural issues, such as 'artificial transcriptional stress responses.' This phenomenon indicates that separation mechanisms could activate the expression of stress-related genes, leading to synthetic changes in cell transcription profiles. This has been confirmed by numerous experimental analyses. An investigation [73] confirmed that the protease separation mechanisms at 37°C could induce the expression of stress-related genes, resulting in practical inaccuracies and potentially leading to imprecise cell type identification.
Following the procedure of converting mRNA directly into the first strand cDNA, the generated cDNA is amplified using polymerase chain reaction (PCR) techniques or test-tube transcription [57]. The cDNA is synthesized from full-length mRNA transcriptomes using a reverse transcriptase with terminal transferase activity. This enzyme, when combined with a second “template-switch” primer, allows for the generation of cDNAs with two universal priming sequences. Once the individual cell-barcoded cDNAs are produced from each isolated cell or single nucleus, they can be sequenced using various high-throughput sequencing techniques [21].
3.3. Single-cell Transcriptomic Sequence Data Analysis
Due to the expanding scope of advanced single-cell RNA sequencing (scRNA-seq) techniques, including clinical specimens, the analysis of these vast volumes of datasets has become a daunting challenge for scientists entering this field of study. Investigating single-cell RNA sequencing (scRNA-seq) datasets has become an increasingly important aspect, and today, it is a primary requirement for advancing the implementation of these advanced techniques in life science and clinical studies. To support the utilization of single-cell RNA sequencing analysis methods, numerous developers have made significant strides. As of May 28th, 2021, nearly 1000 different bioinformatic techniques have been developed and made available [74].
During the preparation of single-cell mixtures, intact cells may undergo processes, such as cell death, membrane damage, or multi-cellular adhesion, due to inevitable natural conditions, experimental activities, and practical challenges. To eliminate gene expression interference from low-quality cells, it is essential to perform additional quality control measures using appropriate techniques, like Seurat [75], Scran [76], and Scanpy [77].
Different studies have shown that Seurat is one of the most widely used techniques with integrated capabilities for purifying poor-quality cells. Primarily, the following quality control (QC) metrics should be used to decide whether a cell should be retained: the number of genes, the number of UMI (transcripts), the proportion of mitochondrial genes, and the ratio of ribosomal protein genes in each individual cell. There is no perfect method for setting the filter threshold, which largely depends on the cell types and tissues being analyzed. Other studies [78] purified cells with less or equal to 100 or greater or equal to 6000 expressed genes, less or equal to 200 UMIs, and greater or equal to 10% mitochondrial genes. In another study [79], standard cells were maintained utilizing the following framework: (1) 200 less than the overall amount of expressed genes per cell (nGenes) less than 2500; (2) 300 less than the full amount of UMIs per cell (nUMIs) less than 15000; and (3) proportion of UMIs tracked to mitochondrial genes (MT%) less than 10%.
Similar to the analysis of conventional bulk RNA sequencing datasets, each cell is treated as an individual sample when analyzing single-cell RNA sequencing datasets. However, the raw expression data cannot be directly used for downstream analysis due to differences in expression levels among cells caused by systemic biases or technical noise, such as variations in sequencing depth and transcript capture efficiency for each cell. Normalization is essential to mitigate technical noise and ensure comparability across all single cells. An investigation [80] assessed the validity of seven normalization techniques, such as BASiCS, GRM, Linnorm, SAMstrt, SCnorm, scran, and Simple Norm.
The scRNA-seq dataset contains a vast number of features, with thousands of cells in a specimen and millions of genes expressed in every single cell. The majority of genes in each cell belong to housekeeping genes, which are detected when there are no significant variations in expression levels within each cell, and their presence can obscure the true biological information. Subsets of genes that exhibit significant cell-to-cell differences in expression levels are known as highly variable genes (HVGs). HVGs not only identify biological stimuli but also greatly accelerate downstream analysis of individual cell RNA sequencing datasets due to the significant reduction in computational burden. A good-quality set of HVGs should include genes that can characterize various cell types, and the quality of HVGs has a substantial impact on the accuracy of cell grouping. Another study [81] assessed seven techniques for characterizing highly variable genes (HVGs), including BASiCS, Brennecke, scLVM, scran, scVEGs, and Seurat. It identified significant heterogeneities in the outcomes and operational times of various methods. Correlation with another tool, Scran, was able to identify a reliable number of HVGs with very good performance and independence from the mean. Brennecke was found to exhibit stable and consistent performance across a wide range of datasets. Scran and Seurat were highlighted as performing the best across a portion of the datasets. Moreover, BASiCS and scLVM_LogVar were observed to be more stable than other techniques.
3.3.1. Data Preprocessing
The fundamental configuration of unprocessed sequencing datasets for single-cell transcriptomes comprises FASTQ and BCL formats, depending on the dataset's origin and sequencing platform. Initially, only FASTQ data can be directly used for standard control. If the unprocessed dataset is not in FASTQ format, the first step is to convert it into FASTQ format using relevant techniques. FASTQ data can be generated from BCL data using cellranger mkfastq, a pipeline that incorporates the banded bcl2fastq program. Specifically, a recognizable CSV matrix dataset with a minimum of three columns (lane, sample, and index) needs to be provided in addition to the path of BCL extracts. Subsequently, FastQC can be executed to assess the quality of the raw single-cell RNA sequencing dataset [21].
3.3.2. Exploratory Analysis
To thoroughly uncover functional errors and biological significance within a given cell population, it is crucial to conduct functional enrichment analyses on a focused set of differentially expressed genes. Global analysis approaches for activity enrichment, such as gene ontology and Kyoto Encyclopedia of Genes and Genomes (KEGG) pathway analysis, are also beneficial for single-cell datasets. Numerous methods for functional enrichment determination have been developed. A study conducted by Huang et al. comprehensively differentiated 68 enrichment analysis techniques in 2009, assessing their robustness and drawbacks [82].
Additionally, GSVA (Gene Set Variation Analysis) is widely applicable in the process of functional enrichment analysis and additional control investigations using a pathway-based approach. GSVA can assess enrichment outcomes for various signaling pathways in each specimen to elucidate the sources of morphological variations, complementing the KEGG pathway analysis and providing more physiologically explanatory outputs [83].
To detect the transcription factors enriched in every one of the cell groups from a single-cell RNA sequencing dataset, a study [84] developed SCENIC (Single-Cell Regulatory Network Inference and Clustering) techniques. SCENIC extrapolates transcription factors by initially obtaining the enrichment of TF motifs through the identification of putative regulatory regions of target genes. Subsequently, TF motif enrichment is used to ascertain the relationship between candidate TF regulatory factors and desired target genes [85]. Though SCENIC can be implemented in both R and Python, pySCENIC is highly recommended for processing large datasets due to its faster execution of SCENIC outputs. It is worth noting that the latest version of SCENIC supports analysis for Homo sapiens, Mus musculus, and Drosophila melanogaster, with the option of manually constructing a custom database for other species [85]. While SCENIC has been widely utilized due to its outstanding adaptability and robustness across a broad range of datasets, it has been criticized for overlooking the dynamic changes in gene regulation systems across different cell types. IRIS3 is an affiliated cell-class-specific regulon reasoning server from single-cell RNA sequencing [86]. In practical terms, IRIS3 has been highly appreciated by scientists lacking substantial programming expertise due to its adaptable web server interface. However, continuous improvement is needed for IRIS3 in terms of reliability and efficiency.
Generally, during the improvement stage of single-cell sequencing technologies, several outstanding problems were solved to increase the accuracy, efficiency, and applicability of the technologies effectively. Some of these addressed problems include enhancing cell isolation and capture efficiency, enabling accurate amplification of low-input specimens, enabling more comprehensive characterizations into cellular divergence, and investigating the huge amount of data generated from single-cell RNA sequencing tools (for instance: a model of dimensionality reduction, clustering, and trajectory inference was developed to extract informative biological insights from vast datasets), Spatial Resolution (enabled the mapping of gene expression patterns within intact tissue sections), and Cost Reduction and Scalability and Detection of Rare Cell Types and Events) [87].
4. SPATIAL SINGLE-CELL RNA SEQUENCING
Every cell within a multicellular organism interacts with its neighboring environment. For instance, stem cells diverge during development primarily through cell-to-cell interaction and consequential signaling, regulated by the relative position of cells within the embryo [88]. The spatial localization of tissues controls the expression of transcription factors linked to divergence, ultimately giving rise to a robust association of cellular organization with their functions [89]. Location-based transcriptomics is the capability to identify the positional information of transcriptional activity within undisturbed tissue, either for sections or individual cells. It encompasses an array of advanced tools that enable scientists to track transcripts, often down to the sub-cellular level, providing an unbiased map of RNA molecules in every part of tissue sections [90].
Single-cell RNA sequencing (scRNA-seq) has proven to be a powerful technique for genomic investigation at the single-cell level. However, a major drawback of this tool is its inability to capture location-related details of the RNA transcriptome. This limitation arises from the necessity to detach tissues and isolate cells, requiring the capture of live cells from entire tissues without inducing pressure, cell death, or cell aggregation [91].
The spatial context is crucial for understanding fundamental issues related to tissue heterogeneity, such as what is expressed in it, which cells express it, and where exactly it is expressed. Location-based transcript determination represents a significant advancement in medical biotechnology, as it allows the identification of molecules like RNA datasets in their intact physical placement within tissue segments, providing spatial context at the scale of a single cell. Moreover, this technique can also be utilized to detect the sub-cellular placement of mRNA units [92]. This method has been adopted and implemented for the determination of mRNA data with great resolution and increased sensitivity [93]. Following the work of Stahl et al. on the spatial alignment of RNA molecules, various methods have been developed, including in situ sequencing mechanisms, fluorescent in situ hybridization techniques, in situ capture approaches, and in silico methods [94]. Even so, spatial transcripts of RNA molecules are classified into two main types [95, 96]: (1) next-generation sequencing (NGS), consisting of positional determination of RNA transcriptome prior to next-generation sequencing, and (2) imaging-based methods together with in situ sequencing-based mechanism, which concern amplification of RNA and their sequencing in a tissue specimen and in situ hybridization-based procedures. The investigation of the transcriptome is executed by utilizing imaging probes that mixture progressively into the tissue target [97, 98].
Elucidating the spatial localization of the mRNA transcriptome enables investigators to uncover cellular diversity in tissues, tumors, and immune system cells, as well as to detect the sub-cellular dissemination of transcripts in various situations. The slogan of spatial transcriptomics is “any target, any region, any sample.” The placement of any designated cell relative to its neighbors and other structures outside the cell can provide fundamental details for explaining cellular characteristics, cell state, and tissue function in biological networks. Position can also identify the ligands to which cells are prone. While endocrine hormones act at observable levels, many other types of signals act on neighboring cells through cell-to-cell communication or soluble ligands acting in the surrounding area. One can design substances that use cell surface-bound sensory receptors and ligand pairs, the mRNA for which can be investigated by transcriptomic mechanisms [99].
These approaches provide key insights into various biomedical sub-disciplines, such as neurology, embryology, carcinoma studies, immune cell investigations, and histological activities. The functioning of each individual cell in multicellular organisms can only be fully understood in the context of its specific position in the body system. An ideal example illustrating the significance of spatial organization is tumor tissue, where cells interact strongly with the neighboring cancer microenvironment to progress into suppressive conditions that inhibit immune cell activity, thereby evading immune cell defense mechanisms and accelerating proliferation to another level [100].
To comprehend the intricacy of biological networks, spanning from various physiological conditions to the pathological progression of infections, it is crucial to understand the significance of each individual cell and their interactions to integrate the complex functions of tissues and organs in the body. Approaches for elucidating these natural processes involve identifying cells present in the tissue and their spatial locations, as well as their interconnections with one another [101].
5. SINGLE-CELL SEQUENCING APPLICATIONS IN BIOMEDICAL RESEARCH
Nowadays, single-cell RNA transcriptomic investigation is rapidly advancing and becoming an outstanding technique for various scientific inquiries across humans, animals, and plants. It enables highly precise and rapid determination of rare cell types and the discovery of new cells in the body. Furthermore, with insights into gene expression at the messenger RNA molecule and protein levels, biotransformation, cell-to-cell interactions, and positional organization, it becomes possible to explore the mysteries of cell configuration and their implications in health and disease conditions. While the initial discovery and application of single-cell RNA sequencing were predominantly focused on animal and, subsequently, human cells, sequencing in the realm of plant science is still in its early stages, with numerous intriguing challenges yet to be overcome [102].
Currently, the application of single-cell transcriptomic sequencing mechanisms remains limited to only a small number of plants due to practical constraints or extremely limited information on cell types and findings in evolutionary biology. Many plant research groups utilize the most widely used model plant in molecular genomics, Arabidopsis thaliana roots, for advanced single-cell transcriptomic sequencing and spatial transcriptomics investigations. This choice is due to the relatively small number of cells, well-identified gene markers, and straightforward techniques for isolating individual cells through enzymatic cell wall degradation mechanisms [103-105].
Analyzing cells at the individual cell level provides a unique opportunity to characterize interactions within inherent cellular processes and external signals, such as regional information or adjacent cells, in cell fate investigations. Dissection at the single-cell level is also of good insight into the clinical diagnosis, assisting in realizing in what way an ‘outlier cell’ may influence the result of the disease process [9], antimicrobial drug adaptation [10, 11], and tumor reversion [12]. The latest technological breakthroughs have made single-cell RNA sequencing an increasingly influential technique for elucidating biology and cellular activity, investigating diseases, predicting treatment responses, and making medication choices [21].
Every tissue and organ is organized into highly structured and functionally diverse groups of cells, which vary depending on different conditions, physiological transformations, divergence routes, and spatial locations. This sophisticated yet well-organized microenvironment maintains equilibrium until extreme situations occur that may transform the normal cell framework into another, such as tumors. To elucidate the development of tumors, the developmental origin of cells, tumor proliferation, malignancy, and treatment responses, it is significant to enhance our understanding of tumor microenvironments with key immunological and stromal components [106].
Single-cell transcriptomic sequencing investigations can analyze functional, healthy cells alongside tumor cells at various stages of tumor growth. This enables accurate outcomes and comparisons between differentiating and determining the efficacy of various medications, leading to the development of more efficient therapeutic strategies for tumors. Initially, single-cell RNA sequencing (scRNA-seq) tools were focused on analyzing every individual region of the tissue, its diversity, and the cell types involved, resulting in the generation of extensive datasets [107].
Many challenges arise from the fact that tumor cells are variably located within tissue organization, resulting in a heterogeneous microenvironmental organization consisting of both tumor and non-tumor cells at various levels and conditions. Furthermore, the combination and distribution of cell specimens can vary even within uniform sections of a tumor, especially if the samples are obtained at different points in time or under different conditions. Additionally, single-cell gene expression level datasets typically contain various sources of noise, leading to cells of the same type being grouped differently and cells of different types being grouped together due to batch effects [108].
Despite the importance of single-cell transcriptomic sequencing, RNA expression profiling does not always provide insights into protein levels or post-translational modifications. Currently, single-cell RNA sequencing investigations are complemented by other methods like mass cytometry (cytometry by time-of-flight, CyTOF). For example, both approaches have demonstrated that regulatory T lymphocyte cells in tumors express higher levels of tumor necrosis factor receptor superfamily member 9 (TNFRSF9; encoding 4-1BB), as well as T cell co-stimulator (ICOS) and cytotoxic T lymphocyte-related antigen 4 (CTLA4), compared to T regulatory cells in blood or adjacent healthy tissue. This observation is indicative of an activated state [109]. Additionally, by incorporating spatial information into single-cell RNA sequencing datasets, we are capable of examining molecular, cellular, and spatial tissue networks, as well as inter-cellular connections between individual cells in situ [110-112].
Single-cell RNA sequencing is also frequently utilized to investigate cellular transitions across various conditions and to track cell trajectories through processes, such as differentiation. Several analytical platforms have been proposed to understand such trajectories. Monocle introduced the concept of “pseudo-time” as a quantifiable measure of “progress through a biological process” and employed methods from mathematical geometry to order cells in pseudo-time based on their transcriptome profile. Once cells are ordered along a trajectory, gene expression dynamics over the course of the underlying developmental path can be assessed to identify key regulators and genes with “switch-like” behaviors [7].
The intrinsic differences in gene expression within cells in single-cell transcriptomic sequencing data can be utilized to infer Gene Regulatory Networks (GRNs) [113]. Typically, genes are clustered into co-regulated “modules” based on similarities in their expression patterns [114].
5.1. Applications in Cancer Research
Single-cell RNA sequencing (scRNA-seq) has significantly impacted various fields of cancer research and revolutionized our understanding of intra-cancer heterogeneity, the tumor microenvironment, malignancy, and treatment resistance. Studies have shown that genetic or genomic variations can lead to cells with diverse genetic and phenotypic traits within cancerous tissues, resulting in highly heterogeneous cancer cells [115, 116]. This elevated level of variability may be connected to the approaches of oncogenesis [117] and malignancy [118-120], so investigators are required to execute a more precise examination of cancer cells.
Conventional sequencing mechanisms can only detect cell masses and generate the average signals within clusters of cells, potentially masking the variability present in cancer cells. Consequently, conventional sequencing techniques may not accurately detect cancer cells. Single-cell RNA sequencing (scRNA-seq) tools can compensate for the limitations of conventional sequencing methods. By tracing individual cancer cells and their microenvironment, scRNA-seq enables the characterization of the variability among cancer cells. Furthermore, scRNA-seq can clarify the cell types present within cancerous tissue and identify specific markers. It can also elucidate processes, such as oncogenesis and proliferation. Consequently, scRNA-seq tools have been extensively utilized in the investigation of various cancers, playing a crucial role in the development of novel diagnostic and anti-cancer therapeutic techniques [121]. For example, scRNA-seq was used to track the T cell immune receptor of colorectal tumors, uncovering subclass grouping, tissue organization traits, cancer variability, and drug-focused gene expression of colorectal tumor T cells.
A possible association or transformation between T cell classes and subclasses distributed throughout tissues was identified. By that year, another study [122] utilized this method to assess the characteristics and correlation of genomic copy number heterogeneity, DNA methylation irregularity, and gene expression alteration during the onset and proliferation of human colorectal tumors at the single-cell resolution and multi-class level.
scRNA-seq tools can detect alterations in gene expression during cancer proliferation. By coordinating individual cell transcriptomic sequencing of neighboring healthy tissues and adenomas at various stages of progression in patients, researchers efficiently exposed genomic variation, clonal configuration, and metabolic instability in the process of tumorigenesis. This provided deep insights into the regulation of cancer development levels [123]. For example, in the transition from familial adenomatous polyposis to adenocarcinoma, Chen et al. observed a moderate epithelial-mesenchymal status, indicating that malignant cells retain epithelial traits while undergoing rapid migration in breast tumors [124].
Another example is a study conducted on treatment-refractory bladder tumor patients. In this investigation, single-cell transcriptomic sequencing was performed to elucidate the cancer microenvironment, which includes immune cells, the extracellular matrix, blood vessels, and other cell types, such as fibroblasts [125]. Similarly, single-cell analysis was conducted on renal cell carcinomas compared to benign kidney tissues. This study provided insights into the biology underlying the progression of renal cell carcinoma and how it responds to treatment [126].
Additionally, single-cell analysis is carried out to understand molecular control points or stimulation targets within cancers and to determine how a patient responds to targeting a specific protein or pathway. For example, genome-wide investigations of DNA are being tracked to detect mutations that can alter one's response to treatment [127]. These mechanisms are essential in situations where patients do not respond to standard therapeutics. Such conditions often occur due to the complexity and variability of infected tissues within individuals. Therefore, there appears to be a promising future for the application of single-cell transcriptomic sequencing technologies for personalized treatments [128].
5.2. Implications in the Area of Immunology
Single-cell RNA sequencing (scRNA-seq) tools can analyze every single immune cell, thereby differentiating distinct classes of immune cells and uncovering novel immune cell populations and their associations. This aids in deciphering the complex immune network and suggests new mechanisms for disease therapy. One study utilized scRNA-seq to determine sub-populations of natural killer cells in the spleen and blood of both mice and humans. This study revealed two distinct characteristics that differentiate blood and spleen natural killer cells [129], and using the correlation of transcripts, the resemblance within the two main subclasses, NK1 and NK2, in organs and species is identified. This investigation provides a comprehensive understanding of the biological nature of natural killer cells and contributes to the transition of animal research into human-associated studies. Another study utilized scRNA-seq to determine several subclasses of dendritic cells and monocytes in the human bloodstream. This study uncovered a novel subdivision of dendritic cells that exhibit characteristics of plasmacytoid dendritic cells but are effective in triggering T lymphocyte cells [130].
In order to investigate immune cells and cytokines during a continuous infection period, the use of single-cell RNA sequencing methods is very informative. One study found that the variability of IL-10-expressing CD4 T cells and the production of IL-10 by a subset of helper cells during different diseases play a significant role in advancing humoral immunity [131].
Single-cell RNA sequencing protocols can investigate immunological cells with elevated variability caused by disease agents, precisely identify the genetic makeup of each immune cell, and help elucidate the intricate workings of the body's immune system [132]. Furthermore, apart from disease-causing agents, age increment can also lead to increased cellular variability among individual cells. A study conducted scRNA-seq on CD4+ T cells in various conditions of young and aged mice and found that aging influences cell transcriptomic dynamics, resulting in an increase in the variability of gene expression levels within immune cells [133].
5.3. Implications in the Gastro-intestinal System and Urinary Tract System
Single-cell RNA sequencing (scRNA-seq) has become an influential technique for researchers in various fields due to its ability to elucidate the diversity and complex cell-class organizations of diverse tissues and cell populations. Studies conducted by Haber et al. established numerous new gut epithelial cell subclasses using scRNA-seq techniques and tracked the expression profiles of gastrointestinal epithelial cells [134]. From this transcriptomic expression profile, the nature of gut cells maintaining homeostasis and responding to disease-causing microbes is described. Another investigator, Gao et al., utilized high-accuracy single-cell transcriptomic sequencing to examine the four digestive organs during human embryonic development and several cells of the adult large intestine, revealing the related gene regulatory processes in the development of these four human digestive organs [135].
5.4. Implications in the Neurology
In the organization of the nervous system, there is dissimilarity among each single neuron because there are specific copy number variations in brain cells [136]. The variability among these neurons creates a challenge in investigating how brain circuits are organized and in understanding neuronal connections. However, single-cell RNA sequencing methods can detect numerous different stages of nerve cells and generate in-depth single-cell traces to elucidate and identify divergent classes of neurons and their associated molecules in the brain [137]. Studies [138] have differentiated sub-classes of mouse and human frontal cortical neuronal cells using advanced single-cell methylation sequencing techniques. They discovered a novel organization of neurons in the human frontal cortex and redefined neuron classes based on the methylation patterns of neurons. Additionally, Lake et al. utilized a new single-cell nuclear sequencing technique to trace the second-generation single cells of an adult brain [139]. It is effective to investigate the importance of principal cell classes and to trace normal human brain cells. Another researcher [140] identified a main subset of cerebellar cells and subclasses beneficial to cerebellar development in a mouse cerebellar development trajectory using single-cell sequencing. These investigations will facilitate future research efforts on cerebellar development, neurobiology, and diseases.
5.5. Implications in the Area of Reproductive and Embryonic Medicine
Single-cell RNA sequencing tools are capable of investigating the importance of a limited quantity of cells, which could be utilized for prenatal identification and facilitated reproduction. By characterizing women's egg cells or embryonic cells using scRNA-seq, it is possible to select safe and healthy embryos for transfer, thereby reducing the birth rate of infants with hereditary genetic disorders and helping to prevent hereditary diseases [141, 142].
Embryonic growth can be viewed as the differentiation process from the early zygote to the fully developed stage. Investigating the early stages of embryonic growth requires techniques that are compatible with small numbers of cells. Single-cell transcriptomic sequencing studies have enabled comprehensive investigations of early mammalian development [143], facilitating a shift from hypothesis-driven to discovery-driven science. In animals, the single-cell RNA sequencing method is adopted to track the cell growth of zebrafish and African cockroach embryos, providing valuable insights into a deeper understanding of developmental biology [144]. Another study [145] traced the genome-wide map of human embryos before implantation using single-cell multi-sequence sequencing techniques. This analysis has applications for examining the sophisticated and highly integrated epigenetic processes in the pre-implantation growth of human embryos. A study by Vento-Tormo et al. [146] conducted a transcriptome analysis of placental cells prior to gestation using single-cell RNA sequencing tools and generated placental cell maps. Through the cell map, three sub-populations of perivascular and stromal cells existing in various decidual layers and decidual natural killer (dNK) cells were established. Additionally, the study detected regulatory reactions that may reduce the immune response of mothers while also uncovering interactions that contribute to the favorable outcome of placental development and reproduction.
Another study [147] uncovered the fluctuation activity and molecular mechanisms of gene expression during the generation of spermatozoa in mice, particularly focusing on alternative splicing patterns using single-cell RNA sequencing tools. The study also identified principal regulators for specific phases of male germ cell growth. Furthermore, the group conducted single-cell RNA sequencing investigations of normal human testicular cells and unhealthy testicular cells. Based on the outcomes, a hierarchical pattern of spermatogonial subclasses, spermatocyte subtypes, and sperm cell subclasses was established, along with specific markers for human germ cells. Additionally, alterations in expression profiles in testicular somatic cells in non-obstructive azoospermia (NOA) patients were identified, which may contribute to the pathogenesis of NOA [148].
6. CHALLENGES IN SINGLE-CELL RNA SEQUENCING TECHNOLOGIES
A comprehensive detection of the transcriptomic landscape of every isolated cell enables us to gain a complete understanding of the interactions of transcripts within single cells. However, single-cell transcriptomic sequencing tools still face numerous obstacles. Generally, current single-cell RNA sequencing (scRNA-seq) techniques have limited capture efficiency. For the reason that only a few fragments of each cell’s transcriptome complement (nearly 10% for numerous procedures) [27] are illustrated in the last sequencing libraries, single-cell RNA sequencing (scRNA-seq) has diminished sensitivity and is incapable of accurately determining low-abundance transcripts [53, 149]. The minimal quantity of input material for single-cell RNA sequencing libraries also leads to elevated levels of technical noise, which complicates data analysis and can mask underlying biological process heterogeneity [57, 150].
Techniques for mapping technical divergence in single-cell transcriptomic sequencing datasets have been suggested [151-153]. However, the majority of techniques utilize the specimen-to-specimen heterogeneity in ERCC read counts to pattern and regulate for technical noise in the individual cell dataset. Thus, they could be utilized solely with trials integrating spike-in controls. Furthermore, these methods assume that the spike-in transcript is considered invariant, like cellular RNA during library preparation. However, naked spike-in RNA does not proceed across cellular lysis and is not sophisticated with ribosomes or RNA-binding proteins. Thus, although spike-in operations function as important indicators of transcriptome occurrence and accuracy in an investigation, there are numerous sources of heterogeneity that remain problematic to regulate in single-cell transcriptomic sequencing protocols.
Another possible source of influence arises from protocols for picking out and capturing individual cells from specimen tissue. While techniques like micro-manipulation or laser dissection mechanisms can pick out individual cells from known positions within a cell mass or tissue, these techniques are labor-intensive or require extensive instrumentation. Major single-cell RNA sequencing mechanisms, along with all the advanced high-throughput techniques currently available, initially separate tissues to form an individual cell suspension, thereby capturing every single cell. However, this cell separation procedure is often nontrivial, and enzymatic treatments used to disintegrate tissues may affect cell viability, potentially influencing the transcriptional status of cells [57]. To prevent biases from enzymatic treatments, one study [154] has established methods for executing RNA-sequencing straightly on individual nuclei without the use of harsh protease enzymatic treatments. Single-cell RNA sequencing (scRNA-seq) tools have progressed remarkably since their inception, improving in terms of both transcriptome quantization and experimental throughput. However, low capture efficiency and high levels of technical noise reduce the accuracy and precision of single-cell transcriptomic sequencing techniques. Consequently, more advanced experimental configurations are emerging to facilitate the elucidation of single-cell RNA sequencing datasets [152].
As an alternative, emerging in situ sequencing techniques are capable of capturing and amplifying RNA within the primary tissue context. However, current techniques can quantify only a few hundred genes per cell. These techniques sequence RNA directly within intact cells; cDNA amplicons are generated and circularized, amplified using a rolling circle amplification mechanism, and then sequenced by ligation in situ using the SOLiD platform [155].
Lastly, the majority of single-cell RNA sequencing (scRNA-seq) literature has focused solely on polyadenylated messenger RNAs. Nearly all published single-cell transcriptomic sequencing protocols select cellular RNA transcripts using poly-T priming techniques, which capture only polyadenylated transcriptomes. Consequently, current techniques are poorly suited to examine non-polyadenylated transcriptomic categories, such as regulatory non-coding RNA or bacterial RNA [156]. Random hexamer priming has been proposed as a procedure to simultaneously capture both polyadenylated and non-polyadenylated transcripts in individual cells. Computationally designed “not-so-random” primers could potentially be used to target both poly(A)+ and poly(A)– species while minimizing the capture of ribosomal RNA [157].
7. FUTURE PERSPECTIVES AND CONCLUDING REMARKS
Analysis of single cells is a promising and rapidly growing field that holds enormous potential to enhance our understanding of fundamental biological processes in life sciences and help us better elucidate the nature and complexity of human disease processes, with the aim of discovering more effective treatments. The single-cell transcriptomic sequencing techniques have proven to be one of the most advancing tools in life sciences over the past 10 years. The advancement in scRNA-seq tools and computational devices has made the tool accessible and usable in nearly all operations within the life sciences field. Another valuable application of scRNA-seq is the creation of single-cell atlases at tissue, organism, and body levels. Due to the advancement in new techniques and implementations, a vast amount of scRNA-seq datasets are predicted to be generated and incorporated into openly accessible databases, facilitating the elucidation of gene and cell implications in health and disease conditions [158].
In the near future, high-resolution maps will empower users to examine existing datasets, bypassing the high costs and time-consuming specimen reprocessing. Microfluidics techniques have now catalyzed a significant shift in experimental designs, and theoretically, various methods, such as combinatorial barcoding, could push the boundaries even further. Since they do not require physical isolation of every single cell, these methods allow for more cost-effective parallel processing of cells, potentially enabling even greater scalability in terms of the number of cells analyzed [159].
Integrating single-cell RNA sequencing techniques with other large-scale genetic testing technologies would significantly enhance the capabilities of these instruments. For instance, an important combinational tool is integrating single-cell RNA sequencing (scRNA-seq) with CRISPR-based genome-wide genetic testing, such as Perturb-seq. This approach allows for the investigation of transcriptional factors by deleting numerous genes with CRISPR. Another example is LinTIMaT, which combines individual cell transcript datasets with mutation datasets for ancestry tracing [160]. With the continual progress of single-cell RNA sequencing and the CRISPR gene editing process, like prime editing [161], more of these kinds of integrational advancements and implications are supposed to be offered to the deeper elucidating of gene and cell purposes in the body system. A huge amount of multi-omics investigations and analyses are proposed to be performed to completely assess the gene regulatory mechanism, its importance, molecules, and interconnections for cell classes in normal tissues or organs and disease states [162].
Following are some of the successes and predicted outcomes of applying scRNA-seq methods in combination with other methods to solve complex disease pathology problems: scRNA-seq combined with spatial transcriptomics and multi-omics profiling has uncovered the heterogeneity of cancer cells, discovering rare cell populations and understanding their contributions in tumor progression and treatment resistance. The integration of scRNA-seq with spatial RNA mapping and single-cell imaging technologies may help understand the spatial organization of cell types within brain tissues and reveal novel disease-associated cell subpopulations, culminating in the discovery of possible therapeutic targets. The integration of scRNA-seq with single-cell proteomics and spatial imaging could give a profound insight into immune cell interactions within tissues and immune dysregulation in autoimmune health issues or cancer, leading to the development of immune therapies and vaccines. The integration of scRNA-seq with spatial transcriptomics and infection models could clarify tissue-specific feedback to pathogens and identify key determinants of disease severity or host susceptibility, shaping the development of antiviral drugs and vaccines [163].
Another promising implication in the near future is the integration of single-cell RNA sequencing (scRNA-seq) technology with routine clinical examination strategies and personalized treatment mechanisms. However, currently, the majority of single-cell transcriptomic sequencing-based clinical trials are still in their investigational stages, mainly focusing on reexamining and enhancing the understanding of disease pathogenesis and identifying diagnostic and therapeutic markers. Despite the remarkable reduction in the cost per cell, the overall cost per sample (including library construction and sequencing processes) remains prohibitively high. This continues to be a significant barrier to the widespread adoption of single-cell transcriptomic sequencing methods as a routine diagnostic protocol.
Another challenge lies in the operation, analysis, visualization, and interpretation of single-cell RNA sequencing datasets. Automated single-cell RNA sequencing (scRNA-seq) data analysis pipelines with user-friendly interfaces, and importantly, ones that can be used by personnel without bioinformatics expertise, are needed to further expand the clinical applications of single-cell transcriptomic sequencing.
CONCLUSION
In recent years, single-cell RNA-sequencing techniques have significantly improved due to the advancement in novel experimental and computational tools, enabling the characterization of transcript distribution in millions of individual cells within complex multicellular organisms. Currently, highly accurate, automated, and cost-effective sequencing tools are continually evolving, promising excellent data quality and increased output with minimal hands-on time. The vast amount of information being generated from current and future studies will have a profound impact on various aspects of life science research. This impact ranges from the development of personalized tumor therapies to a better understanding of antimicrobial resistance and host-pathogen interactions. It also includes uncovering the mechanisms controlling stem cell differentiation, elucidating the early stages of human embryogenesis, and gaining deeper insights into fundamental biological processes.
AUTHORS’ CONTRIBUTION
It is hereby acknowledged that all authors have accepted responsibility for the manuscript's content and consented to itssubmission. They have meticulously reviewed all results and unanimously approved the final version of the manuscript.
LIST OF ABBREVIATIONS
cDNA | = Complementary Deoxyribonucleic Acid |
CNV | = Copy number variation |
CRISPR | = Clustered Regularly Interspaced Short Palindromic Repeats |
DNA | = Deoxyribonucleic Acid |
GSVA | = Gene set variation analysis |
NK | = Natural killer cell |
NOA | = Non-obstructive azoospermia |
PCR | = Polymerase chain reaction |
RNA | = Ribonucleic acid |
scRNA-seq | = Single-cell RNA sequencing |
UMIs | = Unique molecular identifiers |
ACKNOWLEDGEMENTS
Declared none.